
In this example, a balanced subsampling scheme is used to determine the optimal sample size for our model. “A systematic review of Sample-Size Determination Methodologies (Balki et al.) provides examples of several sample-size determination methods. Sample-Size Determination Methodology Explained The first one would be Sample-Size Determination Methodology by Balki et al. It can be completely wrong if you consider transfer learning.įortunately, there are some more robust ways to determine if you have the right amount of data in your training set. Let’s take the rule of 1000 as an example (let’s call it that way).
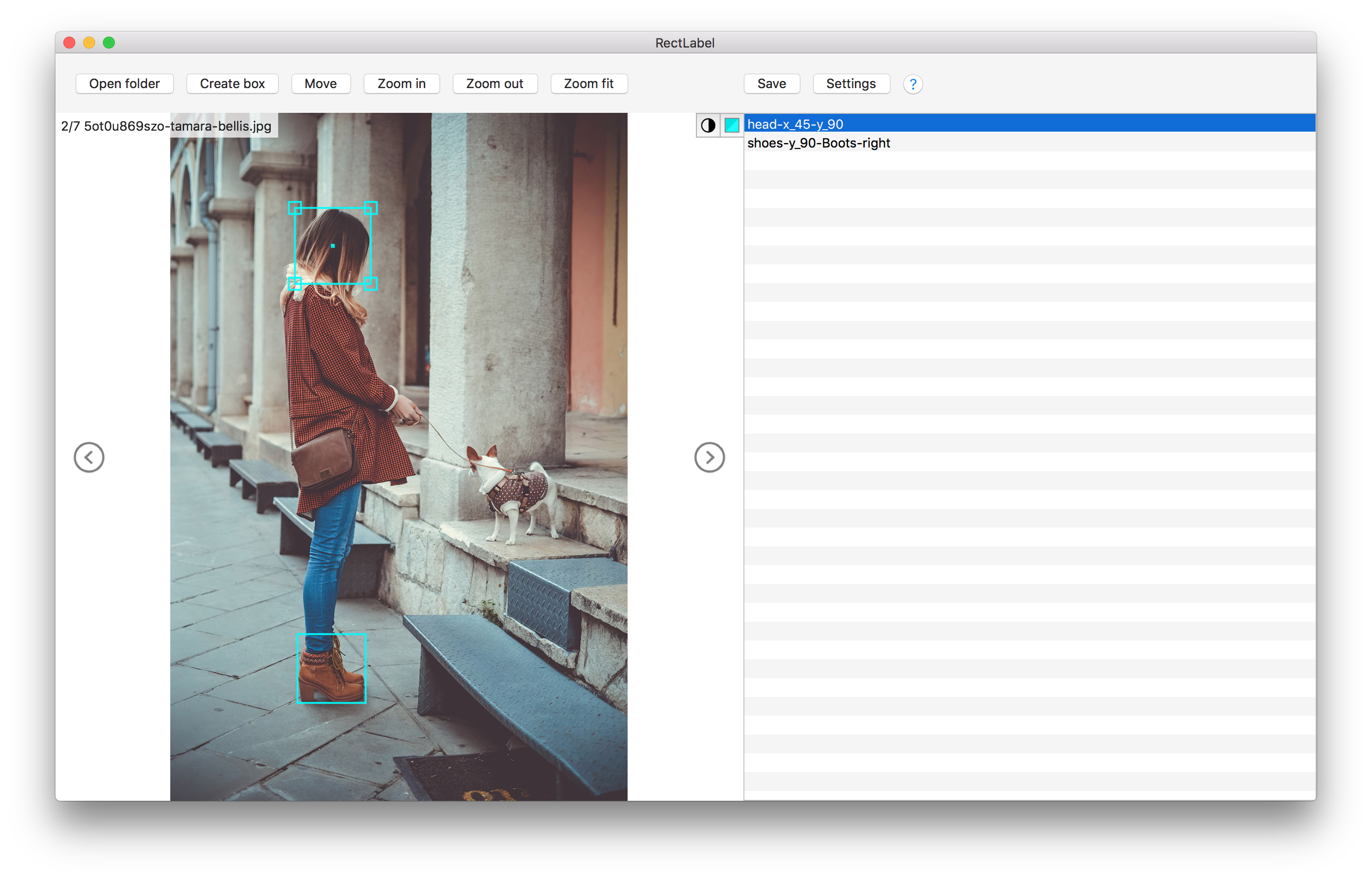
According to one of them, around 1000 examples by class are a decent amount to start with.īut, these types of rules are not strictly “data-science-ish”. However, there are some good rules of thumb that you can follow. It can be complicated to determine the number of images needed in your Image Dataset for an Image Classification task. Empirical Rules to Determine the Minimum Number of Images
Rectlabel price how to#
How to Know if You Have Enough Images in Your Dataset? 1. We’ll be focusing on an image classification as an example. In this blog post, we'll go through these 3 points and how to monitor them in order to optimize the overall quality of your dataset for your use-cases. The relevancy of the images inside the dataset The amount of mislabeled data in your datasetģ. Most of these problems come from the dataset quality itself. Unfortunately, many enterprises are still experiencing issues with their AI models performance. AI companies can now leverage a multitude of tools and services to get their datasets created. This pain led the way for a generation of labeling tools startups and open-source tools such as: In fact, creating an image dataset was truly complicated and time-consuming, and was often done by engineers or interns in a rather inefficient way. For the past years, people have been focusing on model development without investing in training data creation as much as needed.

Every project in Computer Vision starts with a data collection strategy and dataset creation.
